Joint sparse and low rank recovery algorithm for compressive hyperspectral imaging
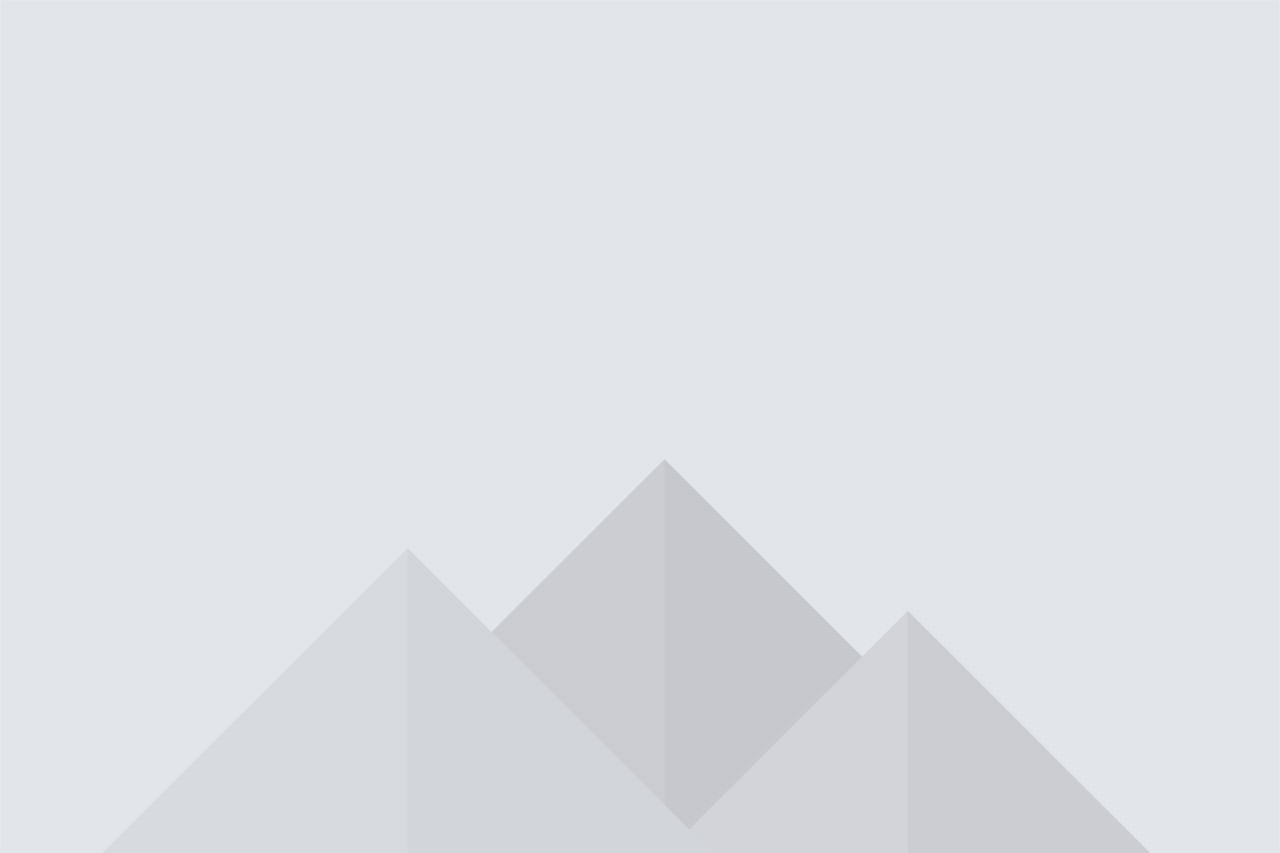
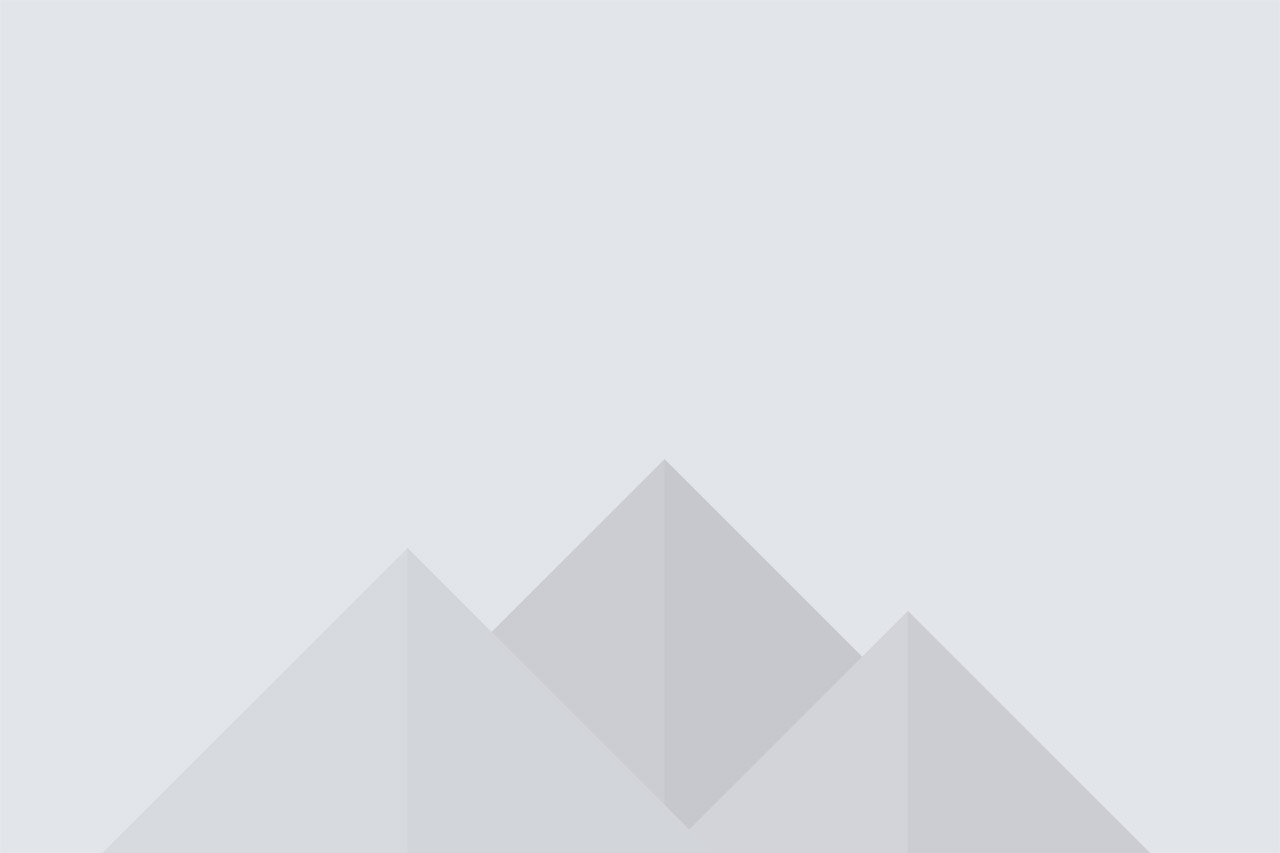
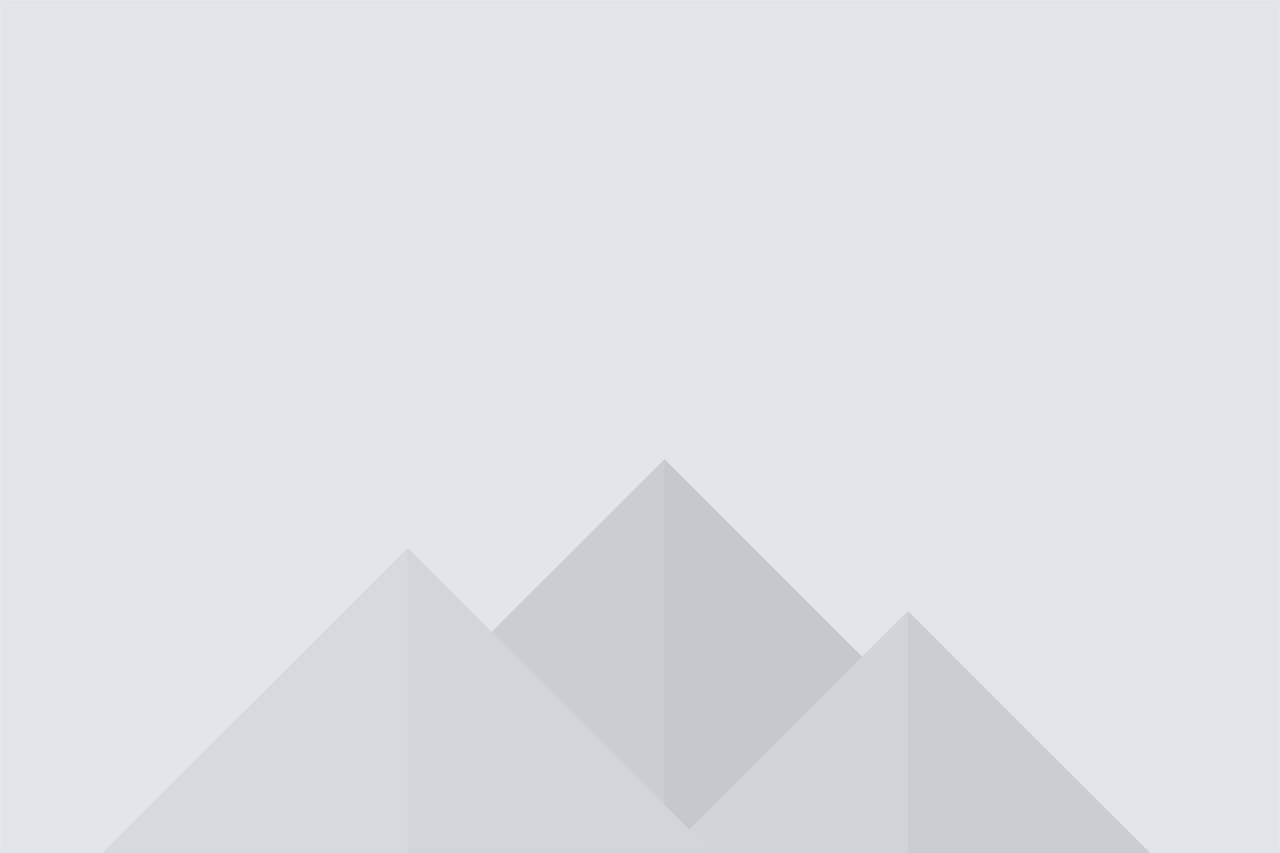
Descripción
2017/8/20
Compressive spectral imaging techniques encode and disperse a hyperspectral image (HSI) to sense its spatial and spectral information with few bidimensional (2D) multiplexed projections. Recovering the original HSI from the 2D projections is carried by traditional compressive sensing-based techniques that exploit the sparsity property of natural HSI as they are represented in a proper orthonormal basis. Nevertheless, HSIs also exhibit a low rank property inasmuch only a few numbers of spectral signatures are present in the images. Specifically, when an HSI is rearranged as a matrix whose columns represent vectorized 2D spatial images in a different wavelength, this matrix is said to be low rank. Therefore, this paper proposes an HSI recovering algorithm from compressed measurements involving a joint sparse and low rank optimization problem, which seeks to jointly minimize the ℓ_2-, ℓ_1-, and ℓ_*-norm …