Interpretable Multiple Loss Functions in A Low-Rank Deep Image Prior Based Method For Single Hyperspectral Image Super-Resolution
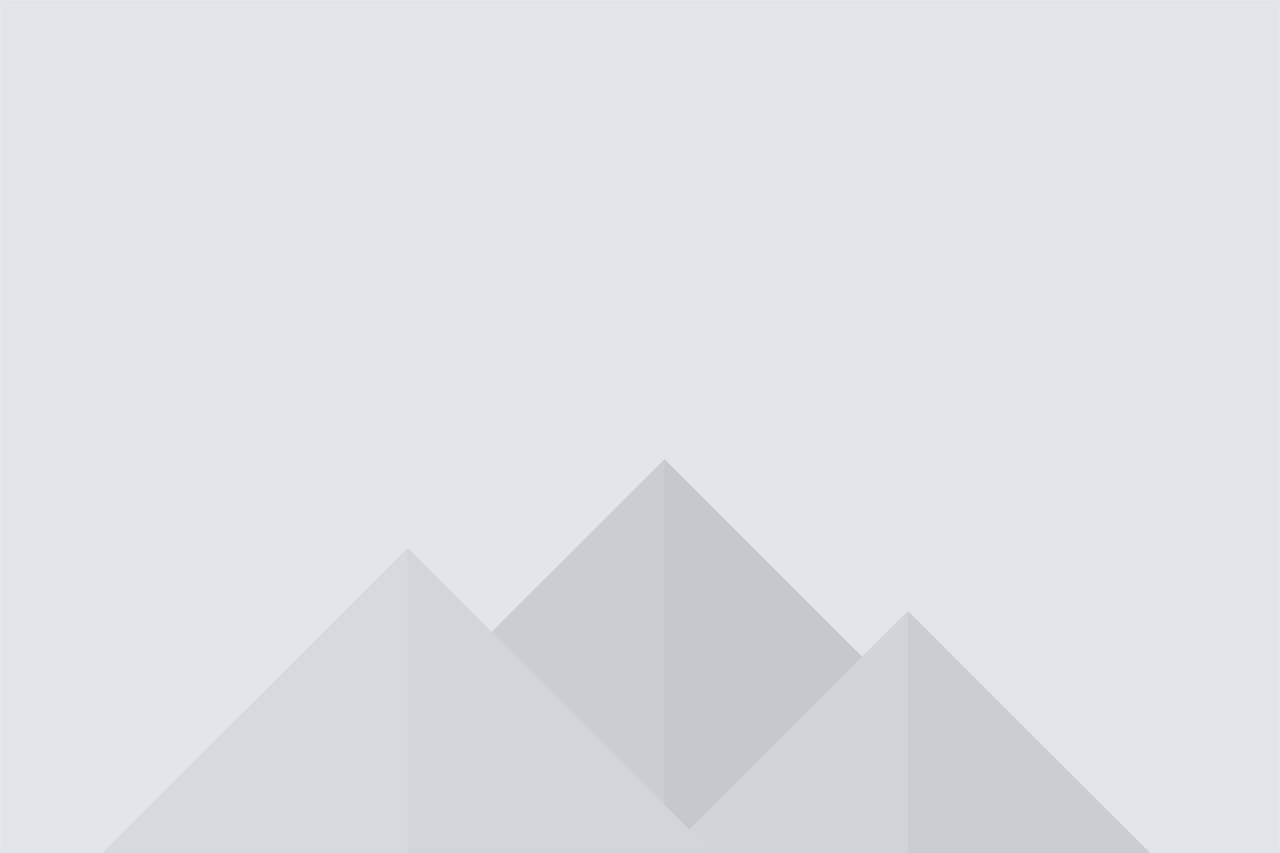
Descripción
2021/8/23
This paper presents a single hyperspectral image super-resolution (HSI-SR) method based on the non data-driven deep image prior approach, where the prior information of the image is intrinsically learned through the weights and the structure of a neural network. Precisely, we propose a scheme composed of a sequence of independent and interpretable deep-blocks, whose outputs are affected in the back-propagation step not only by the general loss-function, but also by a single loss-function, resulting in a scheme using multiple loss-functions. The internal architecture of each deep-block consists of a low-rank decomposition with two block-layers inspired by the linear mixture model, where the spectral image is formed as the product between an abundance and an endmember matrix, such that, the features of the first block-layer and the weights of the second block-layer can be interpreted as the abundances …